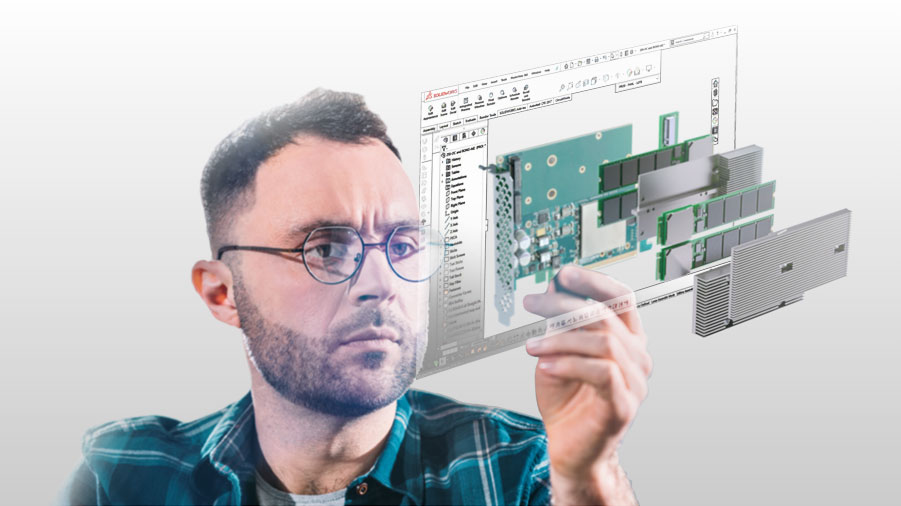
Custom Electronic Product Development, Design and Manufacturing
CUSTOM PRODUCT DEVELOPMENT Design + Manufacturing from BittWare, a Molex Company Custom Product Development Design + Manufacturing from BittWare, a Molex Company Build on our
One of the features of YOLOv3 is multiple-object recognition in a single image. We used this network because it was a more complex, sophisticated approach that would challenge the ability to adapt to a limited edge environment.
Today’s FPGAs are quickly growing in demand for both datacenter and edge environments. This is due to advances in performance, use of easier FPGA development tools, and growing need for the flexibility to tailor hardware acceleration to a specific application. Often working alongside CPUs, FPGA accelerator solutions are part of a heterogenous approach to computing that is focused on flexible application performance fitted to the end-user’s requirements.
In this white paper, we explain just such an application: traffic monitoring using a recent machine learning-based image recognition system (YOLOv3) adapted using OpenCL to the BittWare 520N accelerator board with an Intel Stratix 10 FPGA. The three aspects mentioned as key FPGA drivers are examined: performance versus CPU, ease of development using OpenCL instead of HDL, and the flexibility of tailoring the application (in this variable calculation precision including single-bit binary weights) to use the minimum power/resources.
Until only a decade ago, Artificial Intelligence resided almost exclusively within the realm of academia, research institutes, and science fiction. The relatively recent realization that Machine Learning (ML) techniques could be applied practically and economically, at scale, to solve real-world application problems has resulted in a vibrant eco-system of market players.
However, any news of breakthroughs in machine learning is still to be weighed against the reality that this is a very computationally heavy approach to solving problems, both in the training phase of a dataset and what’s called the inference phase—the “runtime” where unknown input is translated to inferred output. While the training phase for a machine learning application only needs to happen once in the datacenter over an unconstrained time period often extending to hours or days, the live inference must often happen in a fraction of a second using a constrained hardware platform at the edge of a system.
For the machine learning to grow in adoption, inference solutions must be developed that can rapidly implement the latest machine learning libraries in hardware that can be tailored to the application needs.
One approach to reduce the silicon count (therefore power) required for machine learning inference is reducing the dynamic range of calculations. Reducing from 32-bit to 16-bit floating point arithmetic, for example, only slightly reduces the application performance in recognition accuracy, yet can greatly reduce hardware requirements.
What if we went further? This is where FPGAs can excel because as the number of bits required is reduced, even down to a single binary bit, the hardware fabric adapts to only use what is needed. We can use variable precision within a project as well, including use of the hardened floating-point DSP logic blocks on the Stratix 10 FPGA when required. FPGAs allow the designer to have a range of tools to best tailor the hardware to the application requirements.
In fact, our research focuses on performing machine learning using only binary weights: weights are binarized with only two values: +1 and -1. While many image-based machine learning applications use a series of convolution operations collectively called convolutional neural networks (CNNs), this new CNN variant is known more specifically as a Binary Weighted Neural Network (BWNN). It reduces all fixed-point multiplication operations in the convolutional layers and fully connected layers to integer additions.
Richard Chamberlain is System Architect for BittWare. Richard handles OpenCL development projects, having extensive experience working with high-level programming of FPGAs. He regularly advises customers on the best hardware and development approach for new designs or adapting designs based around other system types such as CPUs or GPUs.
Another key component in this research was using the 520N’s OpenCL support to abstract the hardware development process to a software-like tool flow. This allows for the most recent application libraries to be used as the basis for hardware acceleration—reducing the months or longer it would take to target a specific device and ML library using traditional HDL methods (during which newer, better-performing ML libraries might be released). For example, this white paper stems from work we did for the OPERA project on a BittWare Arria 10-based board, the 385A-SoC. Our development team quickly moved the OpenCL code from that device to the much larger and faster fabric of Stratix 10.
Variable precision, use of DSPs for floating-point, and development using OpenCL combine for an application-tailored hardware solution but with software-like development speed.
Processing convolutions within CNN networks requires many millions of coefficients to be stored and processed. Traditionally, each of these coefficients are stored in full single precision representation. Researchers have demonstrated that coefficients can be reduced to half precision without any significant change to the overall accuracy while reducing the amount of storage needed and the memory bandwidth requirements. Most of the pre-trained CNN models available today use partially reduced precision.
However, by using a different approach to the training of these coefficients, the bit accuracy can be reduced to a single bit, plus a scaling factor (ref). During training floating-point coefficients are converted to binarized values that represent whether a value is either greater or less than the mean of all the input coefficients. This can be represented as either 1,0 in binary notation (Figure 1). The output of the convolution is then multiplied by the mean.
Figure 1 : Converting weights to binary (mean = 0.12)
Firstly, binarization of the weights reduces the external memory bandwidth and storage requirements by a factor of 32. The FPGA fabric can take advantage of this binarization as each internal memory block can be configured to have a port width ranging from 1 to 32 bits. Hence, the internal FPGA resource for storage of weights is significantly reduced, providing more space for parallelization of tasks.
The binarization of the network also allows the CNN convolutions to be represented as a series of additions or subtractions of input activations. If the weight is binary 0, the input is subtracted from the result; if the weight is binary 1, it is added to the result. Each logic element in an FPGA has additional carry chain logic that can efficiently perform integer additions of virtually any bit length. Utilizing these components efficiently allows a single FPGA to perform tens of thousands of parallel additions. To do so the floating point input activations must be converted to fixed precision. With the flexibility of the FPGA fabric, we can tune the number of bits used by the fixed additions to meet the CNN’s requirement. Analysis of the dynamic range of activations in various CNNs shows that only a handful of bits, typically 8, are required to maintain an accuracy to within 1% of a floating point equivalent design. The number of bits can be increased for more accuracy.
There are many different networks that could be investigated for BNN applications, and it is tempting to pick one of the many simpler networks such as AlexNet. However, to really understand the effectiveness of FPGAs for BWNN processing, it is better to use a state-of-the-art network, such as YOLOv3. This is a large convolution network with many convolution layers.
YOLOv3 is a deep network, and errors introduced due to fixed point rounding require more bits per addition than smaller networks like AlexNet. The advantage of FPGA technology is the ability to modify the precise number of bits required. For our design, we used 16 bits to represent the data transferred between layers.
Converting to fixed point for the convolution and removing the need for multiplications via binarization dramatically reduces the logic resources required within the FPGA. It is then possible to perform significantly more processing in the same FPGA compared to a single precision or half precision implementation, or free up FPGA logic for other processing.
The YOLOv3 network is a large convolutional network with 106 layers that not only identifies objects, but also places bounding boxes around these objects. It is particularly useful in applications that require objects to be tracked.
Binary weighted networks reduce the accuracy of the YOLOv3 network only marginally if appropriately trained. The following table illustrates the results obtained for the retrained YOLOv3 network.
The average confidence in this image for bicycles was 76%, and for people was 82%. Compare that to the floating-point on the same image, which would achieve 92% average accuracy on bicycles (16% better) and 88% on people (6% better).
To achieve the best performance for the FPGA, it helps to target network features that map best to the FPGA. In this case not only was the network trained for binary weights, appropriate activation types were chosen that mapped efficiently to the FPGA logic.
OpenCL is a popular language used to express parallelism in CPUs, GPGPUs, and FPGAs. Here the Intel FPGA OpenCL compiler has been used to program accelerators targeting the Intel Stratix 10 device. The target FPGA accelerator for this Whitepaper was the BittWare 520N board.
Performance of FPGA designs are dependent upon many factors including but not limited to:
Stratix 10 devices are very large and more susceptible to these problems than previous devices. The following paragraphs discuss some these issues in more detail.
Figure 2 : BittWare 520N FPGA accelerator board
Combinatorial Logic Depth: The Intel OpenCL tools will pipeline designs automatically where possible, inserting the required registering to achieve the best performance possible on a Stratix 10. However, registering is not always possible if there is feedback in the design. This typically occurs when creating complex indexing requirements that have self-dependencies. Therefore, it is necessary to structure code, where possible, to avoid any such pipeline dependencies; otherwise the clock frequency of the design is dominated by these paths.
Fanout: Fanout refers to signals that have a single source but multiple endpoints. This can cause problems for routing tools as there are a finite number of nets available to use on a device. Congestion of these routes requires some signals to be passed around congestion points, resulting in longer paths and ultimately slower clock frequencies. Fanout can be reduced by being aware at coding time of the impact of sharing variables between multiple parts of the design.
Overpopulation: There is a temptation to cram as much logic into a design as possible; however, there will be a point at which the design clock frequency will start to reduce as the device becomes heavily populated. This is caused by routing congestion due to the large number of signals trying to find a route around the FPGA. For large designs to hit high clock frequencies, they must be heavily pipelined and avoid high fanouts.
Memory Bandwidth: It is not always possible to store enough data in local FPGA memory, and data must be written or read from deep external memory. The 520N has 4 banks of DDR4 memory, giving a total memory bandwidth of ~98 GBytes/Sec. Given the size of the S10 device, this bandwidth is not adequate to keep all the neural network layers fed with data; hence input data must be reused where possible. Fortunately, CNN codes permit a lot of data reuse. This problem can be alleviated somewhat using HBM2 versions of the Stratix 10, such as is used on BittWare’s 520N-MX board.
Ultimately most designs are a compromise of the above, and the BNN design described here is no exception.
Figure 3 illustrates the main processing element of the design, the convolution path. Data has been pipelined where possible into 3 accumulation paths. Each convolution block performs a 32×32 binary weight convolution block, where each of the 32 inputs are shared by all 32 outputs. This is of course causing a high fanout but reducing the pressure on external memory bandwidth. By subdividing what would have been a 96×96 convolution into multiple blocks of 32×32, the fan out routing is constrained to within each block, reducing the overall fanout of the design. Input data is passed to each convolution block via OpenCL pipes, permitting the compiler to insert extra registering if required.
The “producer,” “consume,” and “coefficient” kernels shown in Figure 3 pass data from global memory to the different convolution blocks. The consumer block also performs a floating-point activation function on the output.
Table 1 lists the resources required to perform 1024 16-bit accumulations that represent each 32×32 convolution.
The storage required for storing all input and output feature data exceeds what is available on the FPGA device, even when using 16-bit data. Therefore, data needs to be copied from attached global memory to local FPGA memory in batches, which eventually dominates performance once the number of parallel convolutions increase beyond what the global memory can support.
ALMs | Registers | Ops |
---|---|---|
35305 (2%) | 41601 (2%) | 2048 |
Table 1 : Stratix 10 resources for 32×32 convolution matrix
ogic (ALMs) | MHz | Peak TOps | Speed Up versus OpenMP 32 Threads (Xeon CPU D-1587 1.7 GHz) |
---|---|---|---|
536,122 (57%) | 300 | 5.5 | 50x |
The storage required for storing all input and output feature data exceeds what is available on the FPGA device, even when using 16-bit data. Therefore, data needs to be copied from attached global memory to local FPGA memory in batches, which eventually dominates performance once the number of parallel convolutions increase beyond what the global memory can support.
Table 2 provides some statistics for the final compiled design. Note that the Logic also includes the board support package resource required for host communications over PCIe and global memory interfaces.
The next figures provide a comparison of performance in speed and performance per energy used when compared to both Arria 10 and a Xeon CPU. Note that for Stratix 10, even more cores could be utilized for further speed improvements.
The new BittWare 520N-MX board features an Intel Stratix 10 MX device. This FPGA has 3D stacked high-bandwidth memory 2 (HBM2) with 32 user ports offering a combined memory bandwidth of up to 512 GB/s. This extra bandwidth allows different architectures that could help reduce high fanouts in designs and reduce the need for internal buffering for external memory. MX devices should free up more user logic for processing by simplifying the memory arbitration network that can become complex for memory intensive algorithms and allow new bandwidth limited solutions to CNN that were not previously possible.
The flexibility of FPGAs present opportunities for CNN optimizations per individual network that are difficult, if not impossible, to achieve on other technologies. As industry begins to realize the benefits of neural networks and the number of inference applications increase, so will the requirement for networks tailored for different data sets, accuracy, and power.
Fully realizing the wide range of future applications will inevitably require topologies that cannot be completely fulfilled by generic APIs, particularly for computing on the edge. BittWare’s wide variety of FPGA solutions, combined with CNN FPGA optimization expertise, is uniquely positioned to help industry realize the potential of FPGAs for CNN.
CUSTOM PRODUCT DEVELOPMENT Design + Manufacturing from BittWare, a Molex Company Custom Product Development Design + Manufacturing from BittWare, a Molex Company Build on our
Panel Discussion How Today’s FPGAs are Taming the Data Deluge Problem From Gen5 to AI, NOCs to RF at the Edge Watch the recording for
BittWare SDK Software Development Kit for BittWare FPGA Hardware Overview BittWare’s SDK is a cross-platform collection of drivers, libraries, modules, and utilities that aid in
For a data recorder, how many drives are required for a given sustained data rate? Find the answer in this informative white paper.