Accelerate AI Inference at the Edge
Run the same models & workloads you run today with lower latency and greater power efficiency
What Are MERA, DNA, and SAKURA-I?
MERA Compiler Framework:
- MERA compiler and software framework, is a no compromise ultra low-latency solution, which can optimize workloads across heterogeneous systems supported by SAKURA- I & FPGAs.
- Leveraging MERA, means you likely don’t need to re-train your model to take advantage of DNA IP acceleration.
Dynamic Neural Accelerator (DNA):
Neural Processing Engine IP
- Achieves near optimal compute utilization with patented “reconfigurable data-path technology,” enabling the highest utilization of the AI processors computing elements at low batch sizes.
- In combination with the MERA compiler framework, delivers both high throughput and lower latency at lower power on whatever hardware platform it may be powering, SAKURA-I or FPGAs.
SAKURA-I
- A revolutionary new, 40 TOPS, 10W TDP, AI Co-processor chip that’s designed specifically to achieve orders of magnitude better energy-efficiency vs. leading GPUs.
- SAKURA is hardware designed and optimized to run the DNA architecture, allowing it to run multiple deep neural network models together, with ultra-low latency, while preserving exceptional TOPS utilization.
Video
Ultra-Fast Yolov5 Object Detection with EdgeCortix SAKURA-I
Choosing the Right Solution
Want to accelerate your edge AI workloads? There are many options to get started, whether you’re in early-stage development or ready to go to volume production on a complex module.
SAKURA-I ASIC Development Kit
Get the SAKURA-I chip on a low-profile PCIe card that's perfect for benchtop development. Includes MERA Compiler Framework and tools, with the DNA neural processing engine, embedded into SAKURA-I.
FPGA Accelerator Cards
Includes MERA Compiler Framework and tools, the DNA neural processing engine IP, bundled with BittWare's PCIe accelerator cards featuring Intel Agilex 7 FPGAs. Tap here for more details on this solution.
Custom Card or Microelectronics Module
With a power-efficient ASIC, customized cards or microelectronics modules are a perfect fit.
Overview: EdgeCortix SAKURA-I, best in-class energy-efficient Edge AI Co-processor
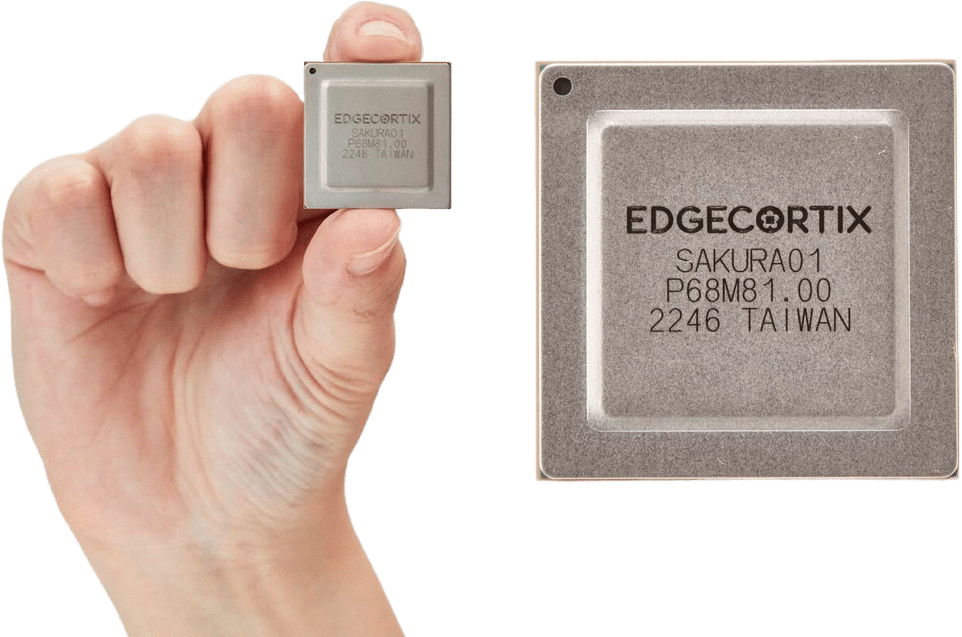
EdgeCortix SAKURA-I is a TSMC 12nm FinFET co-processor (accelerator) delivering class-leading compute efficiency and latency for edge artificial intelligence (AI) inference. It is powered by a 40 trillion operations per second (TOPS), single core Dynamic Neural Accelerator® (DNA) Intellectual Property (IP), which is EdgeCortix’s proprietary neural processing engine with built-in runtime reconfigurable data-path connecting all compute engines together. DNA enables the new SAKURA-I AI co-processor to run multiple deep neural network models together, with ultra-low latency, while preserving exceptional TOPS utilization. This unique attribute is key to enhancing the processing speed, energy-efficiency, and longevity of the system-on-chip, providing exceptional total cost of ownership benefits. The DNA IP is specifically optimized for inference with streaming and high-resolution data.
Hardware Architecture Overview
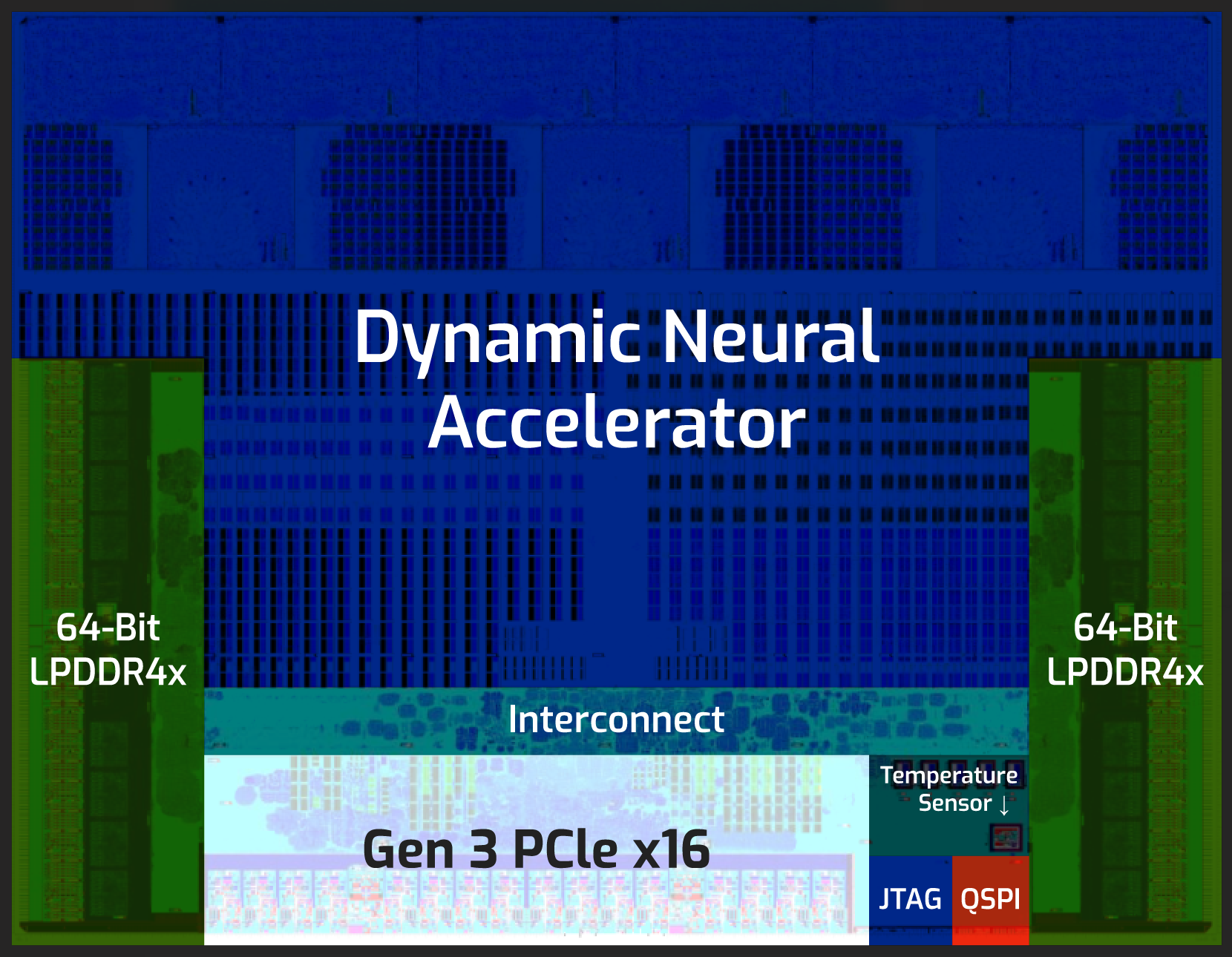
- Up to 40 TOPS (single chip) and 200 TOPS (multi-chip)
- PCIe Device TDP @ 10W-15W
- Typical model Power consumption ~5W
- 2×64 LPDDR4x – 16 GB
- PCIe Gen 3 up to 16 GB/s bandwidth
- Two form factors – Dual M.2 and Low-profile PCIe
- Runtime-reconfigurable datapath
Industries
Automotive
Defense & Security
Robotics & Drones
Smart Cities
Smart Manufacturing
EdgeCortix MERA Compiler and Software
EdgeCortix SAKURA-I AI Co-processor enabled devices are supported by the heterogeneous compiler and software framework – EdgeCortix MERA that can be installed from a public pip repository, enabling seamless compilation and execution of standard or custom convolutional neural networks (CNN) developed in industry-standard frameworks. MERA has built-in integration with Apache TVM, and provides simple API to seamlessly enable deep neural network graph compilation and inference using the DNA AI engine in SAKURA-I. It provides profiling tools, code-generator and runtime needed to deploy any pre-trained deep neural network after a simple calibration and quantization step. MERA supports models to be quantized directly in the deep learning framework, e.g., Pytorch or TensorflowLite.
SAKURA-I Edge AI Platform Specifications & Performance Metrics:
Diverse Operator Support
- Standard and depth-wise convolutions
- Stride and dilation
- Symmetric/asymmetric padding
- Max pooling, average pooling
- ReLU, ReLU6, LeakyReLU, H-Swish and H-Sigmoid
- Upsampling and downsampling
- Residual connections, split etc.
Drop-in Replacement for GPUs
- Python and C++ interfaces
- PyTorch and TensorFlow-lite natively supported
- No need for retraining
- Supports high-resolution inputs
INT8 bit Quantization
- Post-training calibration and quantization
- Support for deep learning framework built-in quantizers
- Preserve high accuracy
Built-in Simulator
- Deploy without the SAKURA-I device, simulating
inference within x86 environment - Estimate inference latency and throughput under
different conditions
Ready for More Info?
Fill out the form to get more information on EdgeCortix AI acceleration solutions.
"*" indicates required fields